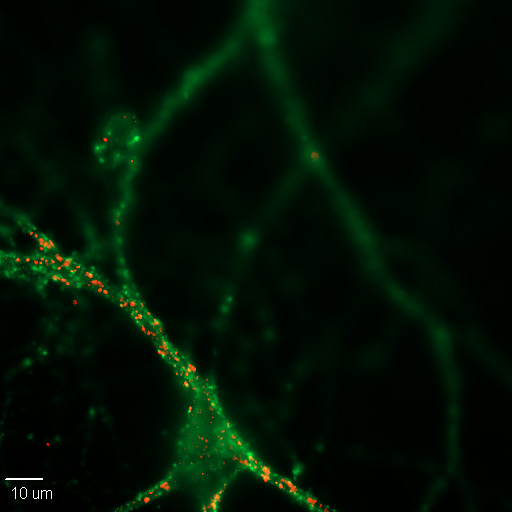
Cambridge (USA) With analog deep learning (ADL), scientists at the Massachusetts Institute of Technology (MIT) have established a new research area within artificial intelligence (AI). The interdisciplinary team is developing a kind of artificial analogue synapses that should enable significantly higher computing power with lower energy consumption in the future.
MIT researchers have already succeeded in creating synapses that outperform the synapses of the human brain by a million. According to a paper from the university, the next breakthrough has now been achieved. The current version of the artificial synapses, which were made from the inorganic material phosphorus silicate glass (PSG), exceed the performance of the previous version by a factor of one million.
“The resistances for analog deep learning are 1,000 times smaller than those in biological cells. Therefore, in the solid state, the space-time-energy performance of the artificial synapses can far exceed that of their biological counterparts,” the scientists explain.
Learning in the human brain occurs primarily through the strengthening and weakening of synapses that connect individual neurons. This approach is technically reproduced in a deep neural network. In this case, the weakening and strengthening of the connections was carried out by training the AI.
The artificial synapses consist of programmable resistors that are comparable to the transistors in processors. A large number of the artificial synapses are then combined into an analogue processor specially optimized for the ADL.
By repeating arrays of programmable resistors in complex layers, researchers can create a network of analog artificial ‘neurons’ and ‘synapses’ that perform computations like a digital neural network. This network can then be trained to handle complex AI tasks such as image recognition and natural language processing.
According to their publication in the journal Science (doi: 10.1126/science.abp8064), the analog processor works in that the resistors allow machine learning by increasing and decreasing electrical conductivity. It is crucial that the inorganic material used can change its resistance in a very energy-efficient manner while remaining unbreakable even at high stresses.
The researchers are also currently working on producing analog synapses using conventional silicon manufacturing techniques. This would significantly reduce the cost of the analog processors and enable industrial production.